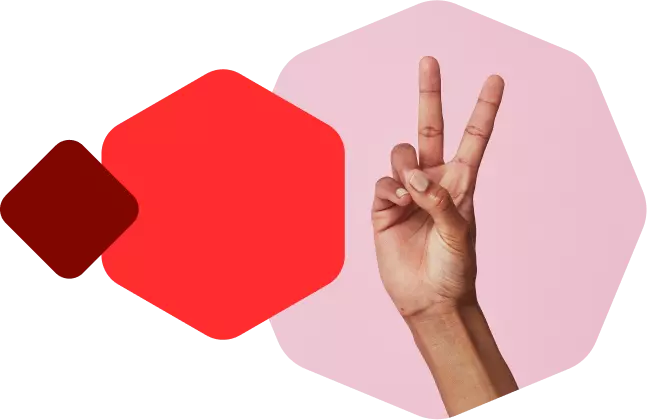
Parked at Loopia
This domain has been purchased and parked by a customer of Loopia. Use LoopiaWHOIS to view the domain holder's public information.
Are you the owner of the domain and want to get started? Login to Loopia Customer zone and actualize your plan.
Register domains at Loopia
Protect your company name, brands and ideas as domains at one of the largest domain providers in Scandinavia. Search available domains at loopia.com »
Get full control of your domains with LoopiaDNS
With LoopiaDNS, you will be able to manage your domains in one single place in Loopia Customer zone. Read more at loopia.com/loopiadns »
Create a website at Loopia - quickly and easily
Our full-featured web hosting packages include everything you need to get started with your website, email, blog and online store.
- Create your website with WordPress
- Start your online store with WooCommerce
- Create your website with Loopia Sitebuilder